The metal body of a smartphone is made of a crystalline material, which has a highly ordered arrangement of atoms. Dropping the phone on its metal back would, at worst, result in a dent. Further, because such crystalline materials have a clear order to disrupt, with potential weak points defined by “defects” in that order, it would be relatively easy to predict which atoms would change places, or become rearranged, to form the dent.
The phone’s glass screen, however, is a different story. It is made of atoms that are jammed together in no discernible order, and dropping the phone on that side could result in shattering. Glass, like many other disordered solids, cannot be deformed significantly before failing, and because such materials lack crystalline order, it is difficult to predict which atoms will shift during failure.
Recently, Associate Professor Daniel Gianola (Materials Department, UCSB College of Engineering) and colleagues at his former academic home, the Department of Materials Science and Engineering at the University of Pennsylvania, collaborated to identify failure criteria shared by seemingly disparate disordered materials. The interdisciplinary team looked at a wide range of disordered solids having constituent particles whose size ranged across seven orders of magnitude.
In an article published in Science, the researchers describe work that incorporates the concept of softness — a counterpart to the defects implicated in the failure of crystalline materials. In a disordered system, softness predicts the location of defects, identifying the collection of particles most likely to change neighbors when the material fails. In this project, softness was used for the first time to establish causal links to defects and plasticity in glasses. Understanding materials failure on this fundamental level could be a key to a range of applications, from designing more-shatter-resistant glasses to predicting geological phenomena, such as landslides.
A dozen of the group’s faculty members, along with students and postdoctoral researchers from their labs, contributed to the study, providing data from fifteen simulations and experiments on different types of disordered systems. The particles in those systems ranged in size from carbon atoms that make up friction-resistant engine coatings to centimeter-sized plastic spheres in a model riverbed.
“This research is emblematic of the best kind of highly cooperative, multidisciplinary effort in which teams made up of people having vastly different perspectives, combine to study a fundamental question that has a large impact,” Gianola said, adding, “Such collaborations are uniquely enabled by centers such as the UCSB Materials Research Science and Engineering Center [MRSEC].”
Referencing the recent, and sixth consecutive, multi-million-dollar renewal of the MRSEC, which, like its University of Pennsylvania counterpart in this study, is a National Science Foundation MRSEC, Gianola said, “I look forward to tackling similarly grand challenges with colleagues at UCSB.”
The researchers used machine learning to reduce hundreds of quantities, each characterizing a specific aspect of the particles’ arrangements — such as their local density or the bonding angle – to a single predictive particle-level quantity that was tied to the propensity for rearrangements. That process produced a value for the microscopic softness, and if softness is known, the team explains in the paper, then the behavior of the disordered material and how likely its constituent particles are to become rearranged can be predicted.
The systems under study were then forced to rearrange as a result of being subject either to random thermal fluctuations or to different kinds of stresses, such as squeezing or stretching. The technique worked equally well in all cases, enabling the researchers to predict with high accuracy the probability that the atoms in a system would rearrange, ultimately causing failure.
Comparing properties across systems, the researchers found that the size of correlated softness was identical to the size of the rearrangements — i.e. the number of particles in motion during the lead-up to failure. Remarkably, they found that this number was nearly identical in every system they studied, regardless of the size of the particles or how they interacted.
Penn researcher Andrea Liu, a team member who has done extensive previous work on disordered materials, said of the project, “For forty years, people have been talking about what determines the size of localized rearrangements. They speculated that there were these localized defects in disordered systems — called ‘shear transformation zones’ — where rearrangements are likely to occur, but no one had seen them, which made predictions impossible. With the machine learning, we’re saying ‘Let's train the system. Let's look at the rearrangements and the structures and see if we can figure out what's important and then use that.’ It’s conceptually straightforward but turns out to be very powerful.”
The researchers also measured the yield strain, which describes the extent to which a solid can be deformed before it starts to yield. Observing systems whose mechanical stiffness spanned thirteen orders of magnitude, they found that the yield strain was approximately the same for all disordered solids. By comparison, the yield strains for different crystalline materials can vary by several orders of magnitude.
“We have always been intrigued by the fact that metallic glasses, despite the heterogeneity of atomic positions and their lack of long-range order, show a fairly constant yield strain, in stark contrast to their crystalline counterparts,” said Gianola. “We wanted to test whether this constant yield strain was a feature observed only in metallic glasses or was, rather, a common feature of all disordered systems, regardless of the type of bonding and size of the constituent particles.”
Knowing that when stress is applied, all the systems look the same up to and around yield, the next step will be to study the dynamics of failure beyond the yield point, at which point chaos ensues and the systems begin to look extremely different.
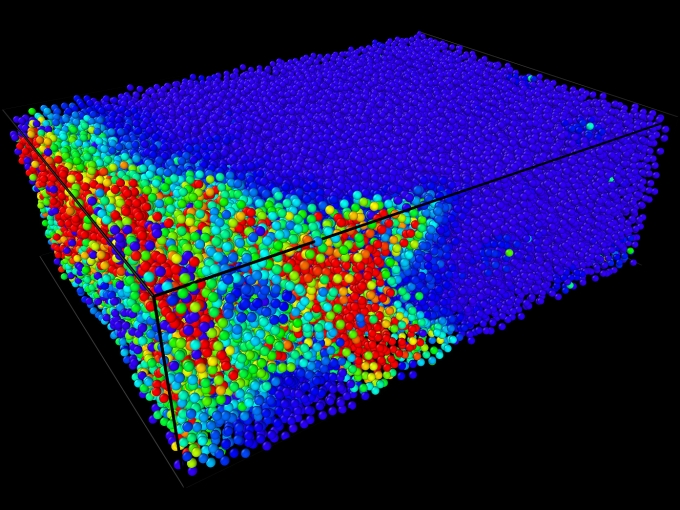
In this snapshot showing a reconstruction of a compression experiment, the red particles indicate hotspots, where atoms are rearranging as a prelude to failure.
Image compliments of Daniel Gianola, Daeyeon Lee, Jyo Lyn Hor, Daniel Strickland