Increasingly, big data and its partner, machine learning, are driving and enabling collaboration. Advances in sensors, cameras, scientific instrumentation, software platforms, deep neural networks, and computing power have made the promise of artificial intelligence real. The results show up in platforms that can identify patterns and scour meaning from millions or even billions of data points to better understand and manage a vast range of dynamical systems, from smart buildings and new materials to human biology and social systems.
Big data can take the form of simple data points that record, say, click-throughs on websites or entries on a spreadsheet, or it can be digital imagery, such as video, photographs, remotely sensed lidar images, or microscopy images. UCSB researchers are on the front lines of this data-fueled revolution, developing systems that make such multimodal big data a powerful tool for engineering.
According to B. S. Manjunath, professor in the Department of UCSB Electrical and Computer Engineering and director of the campus’s Center for Multimodal Big Data Science and Healthcare, big-data approaches require three main elements: experts in the field under study who can frame the research questions and form hypotheses; computational-science experts to design algorithms and data structures; and information-processing experts to address the signaling and information-theory components.
Because so much science-related data takes the form of digital images, UCSB researchers were recently awarded a $3.4 million grant from the National Science Foundation’s Office of Advanced Cyberinfrastructure to fund a broadly interdisciplinary Large-scale IMage Processing Development (LIMPID) project. Their work is based on a platform called BisQue (Bio-Image Semantic Query User Environment), developed by Manjunath’s group. BisQue had its roots in microscopy imaging and was developed to support a wide range of image informatics research for the life sciences. With its ability to process databases and perform image analysis, BisQue makes it easy to share, distribute, and collaborate around large image datasets.
“You can think of BisQue as Google Docs for scientific images,” Manjunath notes. “Imaging data has become ubiquitous, and much of big-data science is image-centric. Working with such data should be as simple as working with text files in Google Docs, so that people can collaborate and share information in real time. Not too many places have that kind of infrastructure for data science. It has taken us twelve years to build, and it’s something that sets us apart.”
BisQue is unique in its ability to handle a wide range of imaging data across diverse scientific applications, from marine and materials science to neuroscience and medical imaging. For instance, recent advances in materials tomography are generating an enormous amount of nanoscale microscopy imaging data, which must be reconstructed, shared, and further analyzed. Manjunath is working with UCSB materials scientist and co-PI Tresa Pollock to integrate algorithms developed specifically for processing materials imaging data into BisQue.
In the field of marine science, most of the billions of images of ocean creatures and habitats that have been amassed to date must be manually processed, according to co-PI Robert Miller, a research biologist in UCSB’s Marine Science Institute. “Until now, we’ve had to look through them and count things, scoring the number of organisms, like algae and fish. And there are millions of these images being taken all around the world every month, if not every week, by scientists, amateurs, divers, you name it.”
But much of that data is going unused. “Even deep-sea survey photos and videos that cost millions of dollars to get are often times just sitting on hard drives, because there’s no willingness to look through it all and get the information out of it,” Miller says. “And there’s not only biological data in there. There’s also data about the seafloor, geology, even archaeology. It’s a huge amount of data that’s being wasted.”
Thanks to the LIMPID/BisQue project, he says, “In the Santa Barbara Channel Marine Biodiversity Observation Network, which is supported by NASA and the Bureau of Ocean Energy Management, we are developing image-analysis pipelines and models to process underwater imagery and automate the processes of identifying and quantifying marine organisms. LIMPID will expand that work to the point where UCSB will become the epicenter of image analysis technology for marine science.”
Meanwhile, at UC Riverside, professor and LIMPID collaborator Amit Roy-Chowdhury will work with neuroscience researchers to analyze large volumes of live imaging data that capture neuronal activities in the Drosophila (fruit fly) nervous system. The UCSB scientists are also collaborating with Nirav Merchant at the University of Arizona, where BisQue and the cyberinfrastructure CyVerse will be leveraged to further enable image-based scientific discoveries. And at the UC San Francisco Center for Digital Innovation, another team, Drs. Rachel A. Callcut and Scott Hammond, have deployed the BisQue platform for use on patient images and associated data. There, BisQue is already serving as a user interface for pixel-level annotation and machine automation of images.
Distinct from the image-based LIMPID project is the U.S. Army–funded Multidisciplinary University Research Initiative (MURI) project focusing on modeling and optimizing team decision-making. CoE computer science professor Ambuj Singh (PI) and professors Francesco Bullo (mechanical engineering) and Noah Friedkin (sociology) are combining their various expertise to model and understand team decision-making and the kinds of interventions that could make teams more efficient. They are using data from multiple sources where decision-making is embedded into systems, such as sports teams, stock-market trading, and small-group surveys.
The ultimate goal, Singh says, “is to move toward teams in which humans and machines work together to solve a specific task. We are already doing that with driving whenever Google Maps tells us which way to go, and we drive there.”
Singh explains that leaders in all kinds of organizations must often form teams that bring diverse skills to bear in solving families of tasks. The MURI collaboration is interested in a series of questions whose answers could help to understand and improve that process, such as: What are the dynamics behind how a team makes a decision? How does a team member learn about the skills that other team members have? Why do some people get decisions right and some get them wrong? What leads team members to change their appraisal of each other in the process, and how does that affect the next round of decision-making the team will face?
“We’re trying to go beyond just a phrase that says, ‘A team is doing a task well or not doing it well,’” Singh explains. “We want to be able to quantify how much better or how much worse something is being done. And we want to know to what extent the entire decision-making process can be captured in terms of a model that can explain the process, and whether it is possible to get assistance from AI in terms of improving this decision-making process itself.”
Clearly, he adds, “You cannot do that with just a single scientific domain alone, so we’re working with multiple faculty members. Francesco Bullo is leading research on the controls and dynamics of the systems, and Noah Friedkin is looking at aspects of social science theory, and how to model the dynamics of groups on different kinds of tasks. I’m there for the computer science and the machine learning.”
The same Army Office of Research that funded the MURI project also greenlighted a parallel project led by Technology Management Program professor and chair, Kyle Lewis (see “New Scientific Method?” on next page), which focuses on other aspects of group learning and, especially, collective intelligence.
The computing center run jointly by the Materials Research Lab (left) and the California NanoSystems Institute faciliatates collaborations around big data.
The MURI grant was awarded with the understanding that the group it funded would support and collaborate with Lewis and her team, leading Singh to quip, “Maybe we are the subjects, and they actually funded our study of groups so that they can study us as collaborating groups!”
These are just a few examples of the many UCSB research projects where big data is a keystone of collaboration.
And on the big-data horizon: a possible campus-wide initiative to integrate data science programmatically into every department on campus. So far, Singh has spoken with over fifty members of the faculty and administration in the run-up to a formal proposal. Now that’s a collaboration.
New Scientific Method?
Big Data Can Subtly Shift How Science is Done
Big data not only serves as a starting point for collaborative research; it can also slightly rewire the traditional scientific method.
For instance, Kyle Lewis, professor and chair of the Technology Management Program (TMP) in the UC Santa Barbara College of Engineering, is collaborating with a related MURI project (see oppostite page) led by computer science professor Ambuj Singh. With funding also from the U. S. Army Office of Research, Lewis is partnering with Singh and his team, mechanical engineer Francesco Bullo and sociologist Noah Friedkin, to study learning behaviors in small groups. Specifically, they are investigating how scientists on an interdisciplinary team “learn to develop shared knowledge so that they can communicate efficiently and work together effectively.” In other words, the project is a collaboration intended to understand and optimize important elements of collaboration.
Lewis explains the process: “We have a theoretical phenomenon that I might understand very well from the social science perspective, and the mathematicians might ask, ‘Can we create a model that not only reflects the theory with fidelity, but also extends it so that we can develop and test new hypotheses about team collaboration?’”
Bullo, Friedkin, and Bullo’s graduate student Wenjun Mei “did the heavy lifting” to develop the mathematical model, Lewis explains, adding, “And once we had a model, we wondered if we could study the phenomenon and replicate it with human beings. So, can we now go into the laboratory with humans and find out if the processes work as we have formally described them as working?
“It’s kind of the reverse of how our science would typically work,” Lewis adds. “Rather than studying human behavior and trying to construct a formal mathematical model based on empirical evidence, you’re developing a mathematical model that is consistent with theories of human behavior and then seeing if you can replicate it in empirical studies. It’s a really exciting way to think about research — using the mathematics to articulate a formal model and then trying to test the model with real people.”
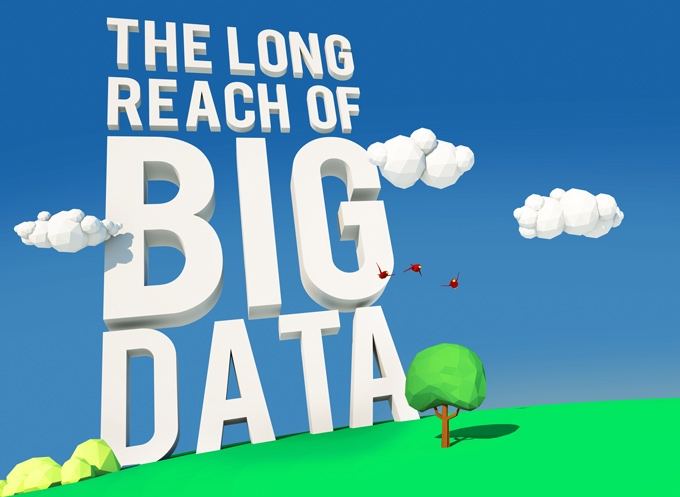