As researchers around the world continue their long and arduous pursuit toward quantum computing, some are working on what might be considered “bridge” technologies to increase energy efficiency by reimagining how a computer carries out computations. One approach is probabilistic computing, and Kerem Çamsari, an assistant professor in the Electrical and Computer Engineering Department in the UC Santa Barbara College of Engineering (COE), has just received a five-year, $546,000 National Science Foundation (NSF) Early CAREER Award to pursue his pioneering research in this rapidly emerging area.
The work, he says, requires “reimagining computers and using them to improve the energy efficiency of power-hungry machine-learning (ML) and artificial-intelligence (AI) algorithms,” adding, “We are very grateful for this support. With this level of sustained funding, we really hope to make a positive impact in making computing sustainable into the future.”
“We are delighted to hear about Kerem Çamsari’s well-deserved NSF CAREER Award,” said Tresa Pollock, acting dean of the UCSB College of Engineering. “His research is important to enabling the “bridging” technology of probabilistic computing, which holds promise for increasing the energy efficiency of the artificial intelligence in its various forms while engineers work toward developing quantum systems. This award further recognizes the tremendous talent, innovation, and leadership of junior faculty in the COE.”
Probabilistic computing is a technological response to the fact that a large class of models in ML and AI are inherently probabilistic. A good example is the recently developed “generative” AI models, for example, chatGPT, which has been much in the news lately. These models output probabilistic results by making guesses at a right answer from a set of plausible answers, providing a different answer even when the same question is asked to the model more than once. (Çamsari describes it as being “a little like talking to a human,” in that you wouldn't use exactly the same words to explain something to someone a second or third time to help them understand it.)
The probabilistic model works as it does because it has access to a "bag" of right answers it can choose from, and it is not necessarily clear which one is "best"; hence, it pays to model the problem (of finding a right answer) probabilistically. On the other hand, traditional computers, based on the silicon transistor, are largely deterministic and precise, and, Çamsari notes, “That precision makes it extremely costly to use them to imitate true randomness.”
Traditional computing is based on deterministic bits, which must have one of two values — 0 or 1 — at any given time. They never fluctuate. They only change as time goes on, according to any specific computation.
A probabilistic bit differs in that it fluctuates constantly between 0 and 1 as a function of time — these fluctuations can be as fast as every nanosecond. It is never a definite 0 or a definite 1, but in a state of constant fluctuation between them. The p-bit is a physical hardware building block that can generate that string of 0's and 1's. That built-in randomness is often useful in algorithms.
Camsari says that researchers in his lab are trying “to design a new fundamental building block,” the p-bit, which is represented by a naturally noisy device. The idea is to design a transistor-like object that uses naturally occurring noise in the environment “to realize the kind of probabilistic behavior we need, starting from the most fundamental building block of the computer.”
To do that, researchers in Çamsari’s lab modify a type of nanodevice used in magnetic memory technology to make it highly “memory-less,” such that it naturally fluctuates in the presence of thermal noise at room temperature. “That provides a steady stream of random bits at practically no cost,”Çamsari says. “Further, because the memory industry has integrated billions of such tiny magnetic nanodevices with silicon transistors, our idea to make them useful for computation is highly scalable. The magnetic nanodevices used by the memory industry are not probabilistic; however, in our work, we are trying to make them lose their memory as fast as possible, and this requires making careful modifications to these devices.”
Çamsari envisions a type of hybrid computer in which such analog p-bits provide true randomness in a classical computer. “You would need thousands of silicon transistors to make a random bit, but the p-bits in this project instead use tiny magnetic units that, unlike transistors, are already noisy and, therefore, consume less power and occupy less space on the chip,” he says. Our projections show that the energy-efficiency and performance of ML/AI models in such a computer will be orders of magnitude beyond what is available with Graphical and Tensor Processing Units (GPU/TPU).”
One challenge of the research is that it is tricky to couple many p-bits together. To sidestep this issue, Çamsari’s team, in collaboration with researchers at Japan’s Tohoku University, have opted to take a hybrid approach, using a single noisy magnetic tunnel junction (MTJ) — essentially a tiny magnet — to drive digital circuits inside what we call a field-programmable gate array (FPGA). This combination of digital, deterministic circuits with noisy p-bits may lead to hybrid probabilistic-classical computers that might extend the capabilities of classical computation.
In a recent publication at the flagship device conference, called the International Electron Devices Meeting (IEDM), Çamsari says, “We gave a tiny example of that approach. We showed how a single noisy MTJ can be integrated effectively with powerful programmable silicon-based microprocessors. In the CAREER proposal, we described a much more ambitious goal of providing an example of this approach one hundred times larger and establishing a regime in which the hybrid computer could significantly outperform stand-alone classical computers.
A key question underlying this research is how the computation of a probabilistic computer compares to that of quantum computation. Çamsari explains: “It is well known that the primary anticipated application of quantum computers will be naturally ‘quantum’ problems, for example, understanding the electronic and physical properties of a complex molecule or uncovering new physics, problems that are too hard to solve using classical computers. Similarly, the primary application for probabilistic computers would be problems that are naturally probabilistic, and we believe that ML and AI are full of such examples.”
Traditional computers cannot solve quantum problems. “If the problem you are trying to solve is quantum mechanical and your computer is not, then you are trying to simulate something,” Çamsari says, before pouring a cup of coffee by way of explanation: “Imagine mixing a cup of coffee with cream,” he says. “Having a classical computer is like trying to simulate that complicated flow by making a model of cream and water molecules and predicting their motion. Building a quantum — or probabilistic — computer is a little like taking a cup, coffee, and cream and then combining them to see how they will mix, with no simulation involved. This is the idea of ‘natural’ computing, in which you build a computer that is naturally inclined by its designed nature to tell you what you wanted to know in the first place.”
Moreover, and importantly, Çamsari adds, “We and others have shown that probabilistic computing can even be used to solve a subset of quantum problems. And while it would not do everything a quantum computer does, probabilistic computing is here now, and a probabilistic computer is easy to build, and, therefore, much easier to scale.”
Çamsari says that his team’s approach “can be viewed as part of a growing trend of developing ’domain-specific hardware and architectures’ — that is, computers designed with a specific domain and a specific purpose in mind, rather than according to the old paradigm of ‘general-purpose’ computers. A key feature is taking inspiration from physics, be it in the form of probabilistic or quantum building blocks. This approach allows computing systems to be built that are natural and energy-efficient. In the case of p-bits, this amounts to harnessing natural randomness in the environment instead of trying to mimic randomness out of precise building blocks.
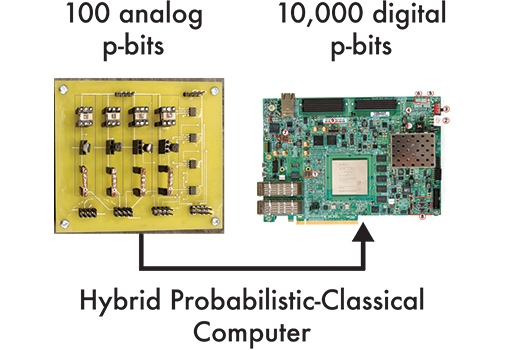
The image shows a hybrid computer where analog p-bits using magnetic nanodevices are combined with digital p-bits that will be used for energy-efficient machine learning discussed in the article.