Big data, machine learning, complex algorithms, sophisticated models, powerful computers — together, these interrelated elements have altered the research landscape. Models are used to simulate polymer self-assembly, the behavior of materials and mechanical systems, processes driving climate change, group decision-making dynamics, agricultural best practices, heating and cooling of skyscrapers, and much more.
Models make it possible to “see” effects that would otherwise be available only by running an impossibly large number of experiments. They save time and money while dramatically increasing insight and understanding. And all models have one thing in common: they require complex mathematics to make them work.
It is the sophistication, the elegance, and the universality of partial differential equations that allow UCSB’s many applied computational scientists to contribute to a remarkably wide range of collaborative research projects.
Some practitioners specialize more than others. Chemical engineering professors Glenn Fredrickson and Scott Shell focus on simulating biochemical processes, such as polymer self-assembly, while materials professor Chris Van de Walle develops theoretical understanding of the quantum-level physics of semiconductors intended for lighting and other energy-efficient electronic applications.
Others cover a wider range of topics. Mechanical engineering professor Igor Mezic is widely known for developing algorithms use to model very large systems, with much of his work falling under the umbrella of what he calls “big data dynamics,” or “using data to analyze and understand the properties of large, data-intensive systems in order to design and control them better.”
In describing one of his operating principles, he highlights the collaborative versatility of mathematicians. “I’m looking for methodologies that can be applied to broad swaths of data across different fields,” he says. “If somebody gives me data from cell biology, I’d like to be able to say something about it with the algorithms I’ve developed previously, without having to change my thinking too much. I want scalability across different types of systems.”
“It often happens that equations you use to describe one phenomenon are very similar to the equations you need to describe or understand a completely different phenomenon,” says materials engineer Anton Van Der Ven in describing the versatility of computational engineers. “Somehow, the way things interact is the same.”
Van Der Ven focuses on the atomic- and electron-length scales to understand why a certain crystal structure is formed from a mixture of elements and to predict useful properties the material might have.
Of that process, he adds, “In developing software tools and methods and collaborating with a lot of people, we recognize that, first of all, some things that we’ve developed can be applied somewhere else, but also things that other people are aware of can help us in solving our own problems. I’m surprised all the time by how other people see a problem.”
In the realm of big data, it is computational scientists who collaborate with others to mine salient truths from a billion data points. “Data does not exist in isolation, but rather as a tool to solve specific problems articulated by subject-matter experts, so experimentalists and applied mathematicians are connected,” says Mechanical Engineering Department chair, Frédéric Gibou. “Computation allows you to play with physics a little bit in a way that would be hard experimentally.”
In terms of the collaborative exchange, Gibou adds, “Experimentalists can tell you things that you can’t find out on your own. In reverse, the experimentalists can ask me to do a simulation on something that might be too expensive for them to run as an experiment or that would require an impossible number of experiments to get some statistics.”
Simulation is also valuable when confronted with a universe of “ifs,” says Linda Petzold, professor of computer science and mechanical engineering. She is currently several years into a large collaborative project funded by the U.S. Army to better understand the process of coagulopathy, a condition in which the blood of a person who is bleeding thins to the point that coagulation, or clotting, stops, putting the patient in grave danger of bleeding out and dying.
Some of Petzold’s collaborators are trauma surgeons, and together, they are examining what is known as the coagulation cascade, a deeply complex process that requires multiple proteins to bind with each other in precise and precisely synchronized ways for coagulation to occur when and where it is needed, and not to occur otherwise.
The system is well known but not well understood, largely because, Petzold says, “It’s so complex that no human being can get their head around it, so it has to be modeled. By running a model, we can simulate things like a blood vessel with blood flow in it and the interactions that occur within the blood, and between the blood and the endothelium, the lining of the blood vessel. We can watch the coagulation develop with computer graphics and test out all sorts of scenarios and ideas about what would happen if this condition or that condition were present.”
Petzold exemplifies the free-roaming ability of the computational scientist. She has built models to simulate the forces on car suspension systems, and in a recent collaboration with UCSB ecologist Cheryl Briggs, she modeled the interactions between frogs and fungus, in an effort to understand the causes of a fungal outbreak that is killing frogs around the world and to identify possible actions to mitigate that disaster.
“If I see an interesting problem that seems amenable to modeling, I’ll go for it,” Petzold says. “The same math applies to many, many different circumstances.”
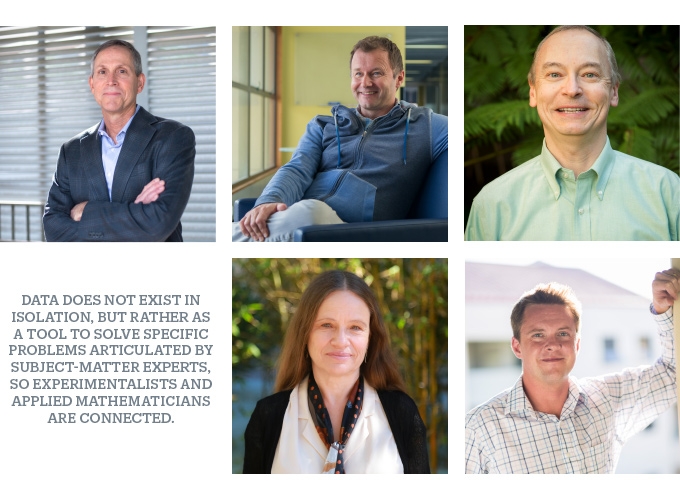
By the numbers (clockwise from top left): Glenn Fredrickson, Igor Mezic, Chris Van de Walle, Scott Shell, Linda Petzold.